
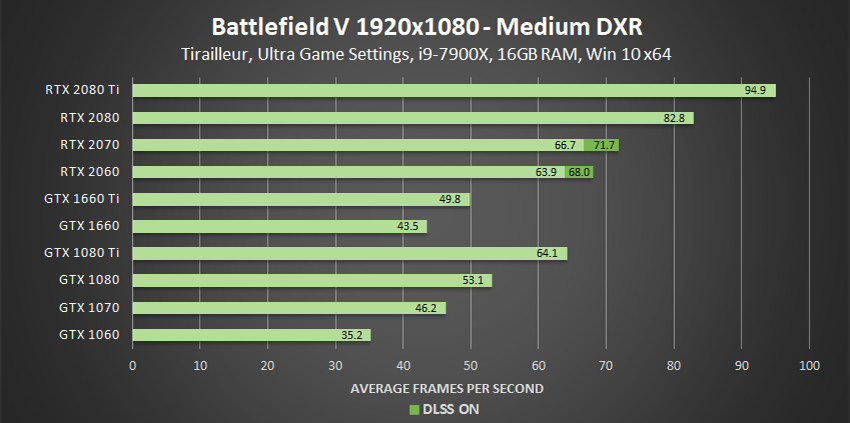
Doing so guarantees consistency between these tasks and reduces the overall computational requirements. The occupancy probability map was trained alongside object detection as an additional task-head of the RadarNet DNN.

Occupancy probability map overlaid with fine-grained semantic segmentation showing the ego vehicle (purple), other vehicles (green), general obstacles (blue), and elevated obstacles (red) Watch an example of the RadarNet deep neural network in action in the NVIDIA DRIVE Dispatch video below.įigure 3. For more details, see NVRadarNet: Real-Time Radar Obstacle and Free Space Detection for Autonomous Driving. More specifically, RadarNet is a deep neural network (DNN) that detects dynamic obstacles and drivable free space using automotive radar sensors. Our system works as part of the ADAS and AV perception in order to detect drivable free space and to further improve 3D perception during multisensor fusion. It is robust against weather and illumination challenges, and is able to directly measure distance. To overcome these challenges, we have developed a free space detection system using radar. However, camera perception performance can suffer in adverse weather and low-light conditions, or when identifying objects at greater distances from the vehicle. Traditionally, camera systems have been used to solve this task. It enables autonomous vehicles to navigate safely around many types of obstacles, such as trees or curb stones, even without being explicitly trained to identify the specific obstacle class.
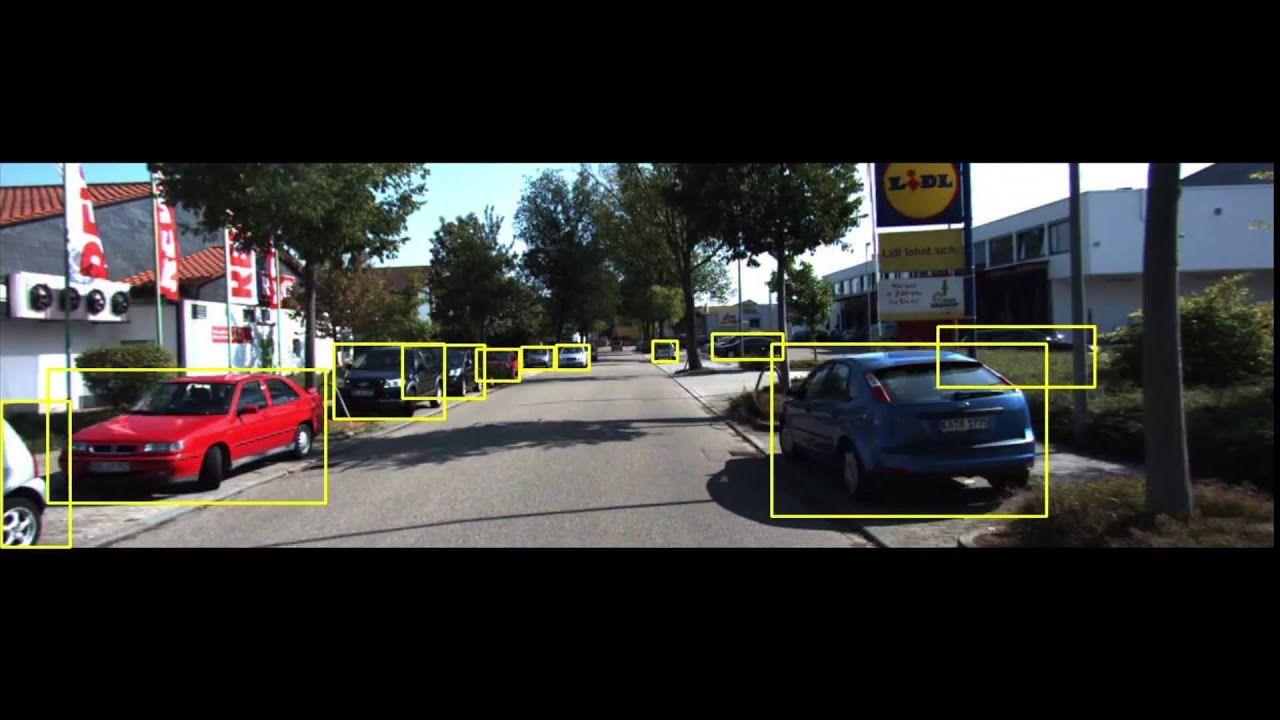
In contrast, free space detection is a more generalized approach for obstacle detection. Obstacle detection is usually performed to detect a set of specific dynamic obstacles, such as vehicles and pedestrians. Detecting drivable free space is a critical component of advanced driver assistance systems (ADAS) and autonomous vehicle (AV) perception.
